A new era of transportation planning is emerging by leveraging a combination of civil engineering, systems engineering, and data science. It is clear that data science is transforming how people work, and its impacts are only increasing with the rapid growth of machine learning (ML) and artificial intelligence (AI). Our team is already looking into how these technologies inform transportation planning for clients and their communities.
New methods of transportation data science eliminate the need to generalize transportation data. Modern data sources, such as connected vehicle data, smartphone devices, commercial fleet GPS hardware, remote sensing, and intelligent transportation systems have significantly improved the quality of transportation planning. With this increased resolution, engineers can evaluate improvements to transportation travel time reliability and identify emerging patterns to inform future design projects.
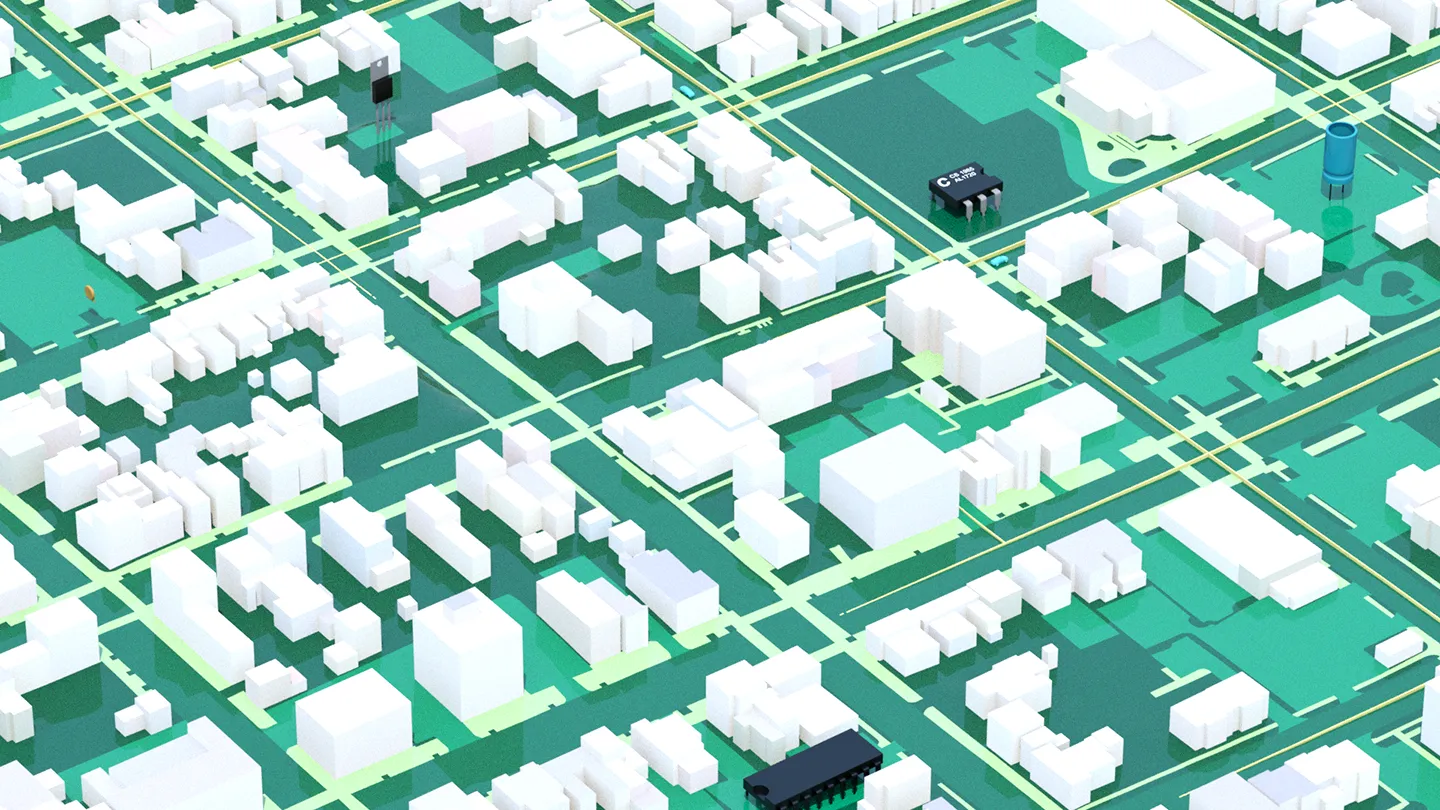
Informing Future Success
Data science allows for project performance to be evaluated through the use of precise observations of its users. With data science, engineers are informed about the conditions that influence traffic operations before, during, and after a transportation project is complete. For example, metrics on congestion, speed of travel, number of crashes, types of crashes, severity, or location-specific events can be used to inform decisions on what should be done differently, better, or maintained on the next project. Better-informed projects help give our clients and communities better outcomes. A disciplined approach to data analytics provides reliable and consistent evaluation methods to instill confidence that decisions are best suited for a specific region and consider multiple criteria, such as safety, environment, and mobility. These criteria allow us to continue improving projects and processes to enhance the value for our clients.
New methods of transportation data science eliminate the need to generalize transportation data. Modern data sources, such as connected vehicle data, smartphone devices, commercial fleet GPS hardware, remote sensing, and intelligent transportation systems have significantly improved the quality of transportation planning. "
Cody Pennetti
Community-Centered Design
Precise data gathered from sources, such as connected vehicle data, allows for design choices that are tailored for unique locations, populations, and clients. Traditional data-gathering methods, such as annual averages and generalized transportation equations, tend to overlook the variability of traffic patterns across hours and days. Modern data science allows for operations that are designed to respond to real-time variable environmental conditions, including unique traffic events, system disruptions, and extreme weather conditions. This allows transportation planners to understand operational differences in communities across specific locations.
Looking Towards the Future
As AI continues to grow and develop over the coming years, so will its functionality. Our engineers are currently using connected vehicle data to uncover insights that aim to use ML and AI to identify system risks. For example, connected vehicles reporting a high density of harsh or autonomous braking events could signify crash-prone intersections and access points before crashes are observed. New technologies and models will provide better information to engineers who will continue to develop new methods of implementation that best support the communities they serve.